Comparing Sentinel-1, Sentinel-2, and Landsat-8 data in the early recognition of irrigated areas in central Italy
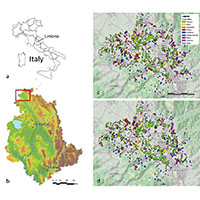
All claims expressed in this article are solely those of the authors and do not necessarily represent those of their affiliated organizations, or those of the publisher, the editors and the reviewers. Any product that may be evaluated in this article or claim that may be made by its manufacturer is not guaranteed or endorsed by the publisher.
Authors
This study evaluated the effectiveness of various remote sensing (RS) data (Sentinel-1, Sentinel-2, and Landsat 8) in the early recognition of irrigated areas in a densely cultivated area of central Italy. The study was based on crop data collected on more than 2000 plots in 2016 and 2017, characterized by quite different climatic conditions. The different RS data sources were used both alone and combined and with precipitation to define corresponding random forest (RF) classifiers whose overall accuracy (OA) was assessed by gradually increasing the number of available features from the beginning of the irrigation season. All tested RF classifiers reach stable OAs (OA 0.9) after 7-8 weeks from the start of the irrigation season. The performance of the radar indexes slightly improves when used in combination with precipitation data, but three weeks of features are required to obtain OA above 80%. The optical indices alone (Sentinel-2 and Landsat 8) reach OA ≈85% in the first week of observation. However, they are ineffective in cloudy conditions or when rainfed and irrigated fields have similar vigour. The most effective and robust indices are those based on combined sources (radar, optical, and meteorological), allowing OAs of about 92% and 96% at the beginning and in the middle of the irrigation season, respectively.
How to Cite

This work is licensed under a Creative Commons Attribution-NonCommercial 4.0 International License.