Hyperspectral prediction of pigment content in tomato leaves based on logistic-optimized sparrow search algorithm and back propagation neural network
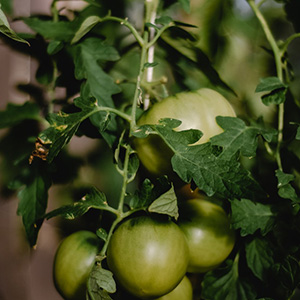
Published:20 June 2023
Abstract Views: 825
PDF: 328
HTML: 9
HTML: 9
Publisher's note
All claims expressed in this article are solely those of the authors and do not necessarily represent those of their affiliated organizations, or those of the publisher, the editors and the reviewers. Any product that may be evaluated in this article or claim that may be made by its manufacturer is not guaranteed or endorsed by the publisher.
All claims expressed in this article are solely those of the authors and do not necessarily represent those of their affiliated organizations, or those of the publisher, the editors and the reviewers. Any product that may be evaluated in this article or claim that may be made by its manufacturer is not guaranteed or endorsed by the publisher.
Similar Articles
- Kai Tian, Jiefeng Zeng, Tianci Song, Zhuliu Li, Asenso Evans, Jiuhao Li, Tomato leaf diseases recognition based on deep convolutional neural networks , Journal of Agricultural Engineering: Vol. 54 No. 1 (2023)
- Wei Wang, Yuanjuan Gong, Xuewei Bai, Rui Tan, Real-time straw moisture content detection system for mobile straw granulator , Journal of Agricultural Engineering: Vol. 55 No. 2 (2024)
- Fan Cui, Guoqi Dong, Baiping Chen, Penglin Yong, Suping Peng, Application of ground penetrating radar technology in moisture content detection of stored grain , Journal of Agricultural Engineering: Vol. 54 No. 1 (2023)
- Zhongju Wang, Chao Huang, Long Wang, Parameter estimation of soil water retention curve with Rao-1 algorithm , Journal of Agricultural Engineering: Vol. 53 No. 2 (2022)
- Guanglai Wang, Congcong Wang, Dayang Liu, Detection of early collision and compression bruises for pears based on hyperspectral imaging technology , Journal of Agricultural Engineering: Vol. 55 No. 4 (2024)
- Hongbo Wang, Zhicheng Xie, Yongzheng Yang, Junmao Li, Zilu Huang, Zhihong Yu, Fast identification of tomatoes in natural environments by improved YOLOv5s , Journal of Agricultural Engineering: Vol. 55 No. 3 (2024)
- Ernest Ekow Abano, Hai Le Ma, Wenjuan Qu, Thin-layer catalytic far-infrared radiation drying and flavour of tomato slices , Journal of Agricultural Engineering: Vol. 45 No. 1 (2014)
- Eliseo Roma, Pietro Catania, Mariangela Vallone, Santo Orlando, Unmanned aerial vehicle and proximal sensing of vegetation indices in olive tree (Olea europaea) , Journal of Agricultural Engineering: Vol. 54 No. 3 (2023)
- Ameneh Sobhani, Shawkat B.M. Hassan, Giovanna Dragonetti, Raffaella Balestrini, Mauro Centritto, Antonio Coppola, Alessandro Comegna, Comparing actual transpiration fluxes as measured at leaf-scale and calculated by a physically based agro-hydrological model , Journal of Agricultural Engineering: Vol. 54 No. 3 (2023)
- Kamalesh Kanna S, Kumaraperumal Ramalingam, Pazhanivelan P, Jagadeeswaran R, Prabu P.C., YOLO deep learning algorithm for object detection in agriculture: a review , Journal of Agricultural Engineering: Vol. 55 No. 4 (2024)
You may also start an advanced similarity search for this article.